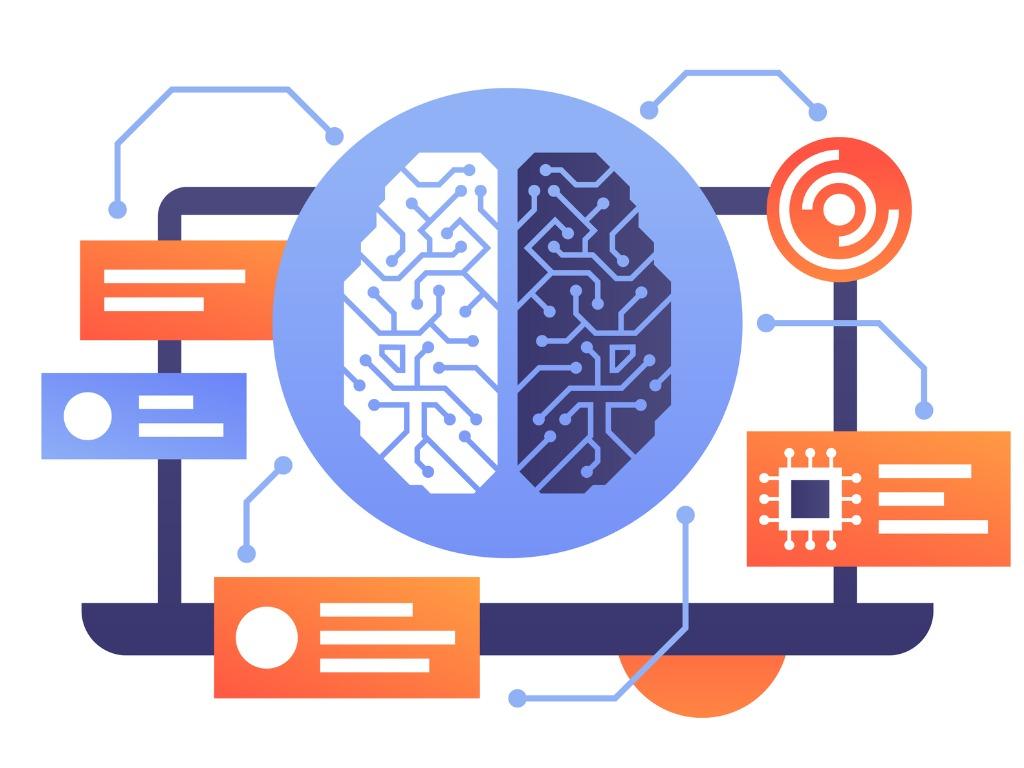
Machine Learning at the Edge: Harnessing Data Processing Power
In a world of massive data sets and complex algorithms, machine learning is becoming increasingly important for solving many of the technological challenges we face. As the technology has advanced, the move towards machine learning at the edge is growing in prominence. Harnessing the data processing power of the edge nodes can revolutionize machine learning applications and provide faster, more detailed insights. This article will explore what machine learning at the edge is and how it can be used.
1. Introduction to Machine Learning at the Edge
For many organisations, the amount of data they are managing is increasing exponentially. To help them process and leverage this data, they must consider the use of Machine Learning at the Edge. By bringing machine learning to the edge, organisations can benefit from enhanced data processing power as well as reduced latency.
The edge is essentially where an organisation’s data processing infrastructure is physically located. By bringing machine learning processing power to the edge, organisations can benefit from a variety of powerful advantages that make it easier and less expensive to leverage data.
- Speed: With edge computing, data is processed in real-time instead of in batches.
- Data Sovereignty: As data stays within an organisation’s infrastructure, security is improved as data is processed faster and more locally.
- Flexibility: Edge computing gives organisations the ability to customise their own data processing infrastructure.
When it comes to implementing Machine Learning at the Edge, organisations must consider the need for both high computational power and specialised algorithms. A high level of computing power is needed to handle the complexities of machine learning algorithms. Such algorithms are often built for specific applications and hence must be tailored specifically for the job at hand.
Organisations looking to implement Machine Learning at the Edge must also pay attention to the design of the infrastructure. They should consider the types of data that need processing, the scale of operations that will be required and the environment where the data will need to be processed. This will ensure that organisations can effectively and efficiently leverage Machine Learning at the Edge to gain insights from their data.
2. Types of Machine Learning at the Edge
The edge is a powerful platform for harnessing data processing power. Machine learning at the edge refers to the application of machine learning models to data that is collected and processed at the edge of a network. This technique enables organizations to rapidly gather data and quickly build models that can be used for predictive analytics and other tasks with minimal latency.
The two main are cloud-based and standalone:
- Cloud-based machine learning at the edge: Cloud-based machine learning relies on servers and computing resources in the cloud. This type of machine learning usually involves data that is collected from various IoT sensors and devices, such as smart cameras. For example, an organization could use cloud-based machine learning to generate real-time insights about traffic congestion.
- Standalone machine learning at the edge: Standalone machine learning is a type of machine learning that is run on computers and devices at the edge of the network, such as a router or an IoT sensor. This type of machine learning is often used for real-time applications such as predicting demand for products or optimizing customer journeys. For example, an organization could use standalone machine learning at the edge to identify customer trends and automatically optimize marketing campaigns.
Both offer powerful capabilities for harnessing data processing power. Depending on the requirements of the organization, one type or another may be more suitable for the task at hand. Organizations should carefully consider their use case when deciding which type of machine learning to implement at the edge.
3. Benefits of Leveraging Data Processing Power with Machine Learning
Harnessing Data Processing Power
- One of the greatest benefits of using machine learning for data processing is its ability to quickly and accurately identify patterns in data. This helps organizations make informed decisions based on available and relevant data points.
- This data can then be used to identify potential areas of growth or uncover opportunities to increase efficiency. In addition, machine learning can help uncover hidden risks or trends that were not previously known, allowing organizations to take advantage of these insights before a competitor does.
- Another advantage of utilizing machine learning for data processing is that it can help organizations get the most out of their data. With the ability to define rules and set parameters, the algorithms leveraged for data processing can be optimized to capture the most relevant information.
- This not only enables organizations to gain insight into previously hidden correlations or patterns, but it can also help drive more informed decision-making and improvements in process efficiency.
- Additionally, machine learning for data processing can help organizations reduce costs associated with data storage and handling. By leveraging machine learning algorithms, many of the mundane tasks related to data storage and handling can be done automatically.
- This means less manpower is needed to manage the data, allowing organizations to focus their resources on more meaningful tasks and utilize their budget more efficiently.
- Finally, leveraging machine learning for data processing will enable organizations to get the most out of their data. Machine learning algorithms are able to quickly and accurately process large amounts of data, which in turn provides insights and helps drive efficient decision-making.
Overall, the advantages of leveraging machine learning for data processing are clear. Machine learning can not only make data processing more efficient and cost-effective, but it can also provide organizations with insights that were previously hidden in the data. With so many advantages, collecting and leveraging data through machine learning is a critical step in becoming a data-driven organization.
4. Challenges in Implementing Machine Learning at the Edge
Harnessing data processing power at the edge has its challenges. This post will cover the common challenges faced when implementing machine learning at the edge.
1. Variations in Hardware Configurations
Machine learning at the edge depends on the device hardware and its configurations. Different devices have different operating systems, memory, storage availability, and processing speeds. This poses difficulties when designing deep learning models for specific devices and scenarios.
2. High Upfront Costs
Getting started with machine learning at the edge requires significant upfront costs. This includes buying the device, network fees, storage, and other charges. For organizations whose budget is limited, this can have a huge impact on the rolling out and deploying of services.
3. Difficulties in Generating Data Labels
Data labels form an important part of the machine learning process. If the data labels are not accurate, then the prediction results from the models will be flawed. Despite advances in machine learning libraries and tools, generating accurate data labels still remain a challenge when implementing machine learning at the edge.
4. Security Concerns
Due to the sensitive nature of data that needs to be collected for machine learning, security concerns are a valid issue to consider. Additional measures need to be taken to ensure the privacy and security of the data being processed, stored, and transmitted at the edge.
5. Guidelines for Successfully Implementing Machine Learning at the Edge
Edge Device Compatibility and Interoperability
- Understand the device class and environment for machine learning at the edge – whether mobile, IoT, wearables, or other types of edge devices, all machine learning at the edge must be device-compatible and work in the intended deployment environment, according to specific requirements.
- Ensure both the machine learning model and its infrastructure are compatible and interoperable with the edge device, to maximize performance and reliability.
Data Collection and Cleansing
- Establish means to collect datasets from a variety of sources, such as mobile devices to cloud-connected IoT devices.
- Cleanse the datasets of any potential inconsistencies or errors, to ensure machine learning models learn accurately from the data.
Algorithmic Selection and Training
- Carefully select the best-suited algorithms to efficiently process the data, to leverage the benefits of both supervised and unsupervised learning.
- Properly train all the components of the model accurately, to ensure optimal results with minimal overhead cost and development time.
Security and Privacy Considerations
- Thoroughly consider data privacy and security in the process of developing and deploying machine learning at the edge, ensuring the required security protocols and frameworks are in place.
- Keep an ongoing awareness of potential security threats and mitigation strategies to safeguard the data in the edge device.
Hardware Limitations
- Understand the available hardware capabilities of the edge device and tailor the machine learning model according to its specifications, to make the most of the available resources.
- Incorporate techniques such as frugal deep learning to reduce the overhead costs associated with edge device processing.
Q&A
Q: What is Machine Learning at the Edge?
A: Machine Learning at the Edge is a new technology that enables data processing tasks to be performed at the edge of a network, such as on a mobile device or IoT device.
Q: What advantages does Machine Learning at the Edge provide?
A: Machine Learning at the Edge provides advantages such as improved safety, privacy, and scalability, as data processing can be done without having to send it off to a cloud-based server.
Q: What challenges come with implementing Machine Learning at the Edge?
A: Challenges associated with Machine Learning at the Edge can include device power and compatibility, as well as data privacy and security considerations.
Q: What kind of applications can benefit from Machine Learning at the Edge?
A: Machine Learning at the Edge can be applied to a wide range of applications, such as robotics, autonomous vehicles, healthcare analytics, security and surveillance, and smart cities.
Q: What skills do I need to implement Machine Learning at the Edge?
A: Skills that are useful for implementing Machine Learning at the Edge include knowledge of programming languages such as Python, as well as knowledge of embedded systems and the ability to create and train machine learning models.
Q: What different types of machine learning are used with Machine Learning at the Edge?
A: Machine Learning at the Edge commonly uses supervised and unsupervised learning techniques, as well as deep learning methods.
Q: What tasks can Machine Learning at the Edge help with?
A: Machine Learning at the Edge can help with tasks such as real-time or near-real-time analysis, object recognition, facial recognition, classification, and natural language processing (NLP). In conclusion, machine learning at the edge is a potential game-changer for data processing. As technology advances, edge computing provides opportunities for businesses to become more efficient in their data workflow, while providing customers with a seamless and quickly-accessible service. With a wide variety of applications, this technology is a powerful tool for businesses to increase efficiency and create products that can benefit consumers.